The generation of large amounts of data, which must be properly managed, represents one of the main issues in collider experiments. In one year of operation, approximately 40k ExaBytes of raw data are produced at the Large Hadron Collider
(LHC) at CERN [1]. By 2026, it is believed that the amount of data produced will equal 800 PB per year, thanks to the High Luminosity LHC (HL-LHC) upgrade. The raw data are currently filtered by ATLAS and CMS (the two main general-purpose
particle detectors at the LHC) using a two-step selection process [2]. The selection process of relevant events, known as triggering, allows for storing salient data for later analysis. A significant problem is making sure that
important information is not discarded, and the ever-increasing amount of data produced makes it hard to maintain existing trigger schemes.
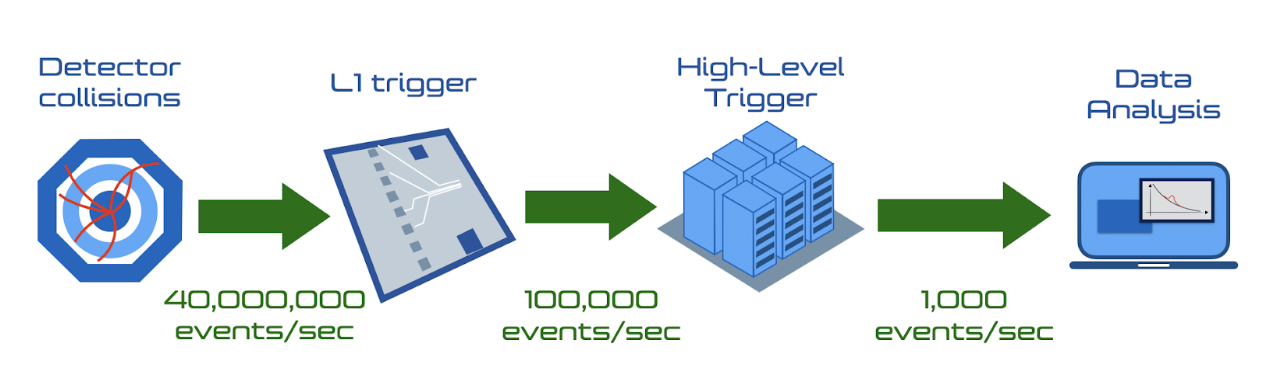
Typical data flow in the ATLAS and CMS experiments, with raw data filtered using a two-step selection process.
Help in this sense can come from Deep Learning algorithms, which have proven to be extremely useful and effective when dealing with large amounts of data. The ability to execute Deep Neural Networks at the trigger level to improve online selection performance will be crucial for current and future high-energy physics experiments. Low-latency hardware solutions exist, e.g. FPGAs, but the primary constraint to the implementation is often related to the model’s size, which has to be finely tuned not to exceed the available memory. We investigate novel approaches to reduce the size of models, having under control the model performances [3, 4].
[1] Clissa L., Lassnig M., Rinaldi L. How big is Big Data? A comprehensive survey of data production, storage, and streaming in science and industry. Published in: Frontiers in Big Data, 6 (2023).
https://doi.org/10.3389/fdata.2023.1271639
[2] Smith W. H. Triggering at the LHC. Published in: Ann. Rev. Nucl. Part. Sci., 66, 123–141 (2016).
https://doi.org/10.1146/annurev-nucl-102115-044713
[3] Di Luca A. , Mascione D., Follega F. M., Cristoforetti M., Iuppa R., Deep Neural Network resizing for real-time applications in High Energy Physics, Published in: PoS LHCP2021 (2021), 257, DOI: 10.22323/1.397.0257
[4] Mascione D., Cristoforetti M., Di Luca A., Follega F. M., Iuppa R. Innovative deep neural networks resizing for FPGA implementation in future collider experiments. Published in: Nuovo Cim. C, 46(4), 97 (2023).
https://doi.org/10.1393/ncc/i2023-23097-6